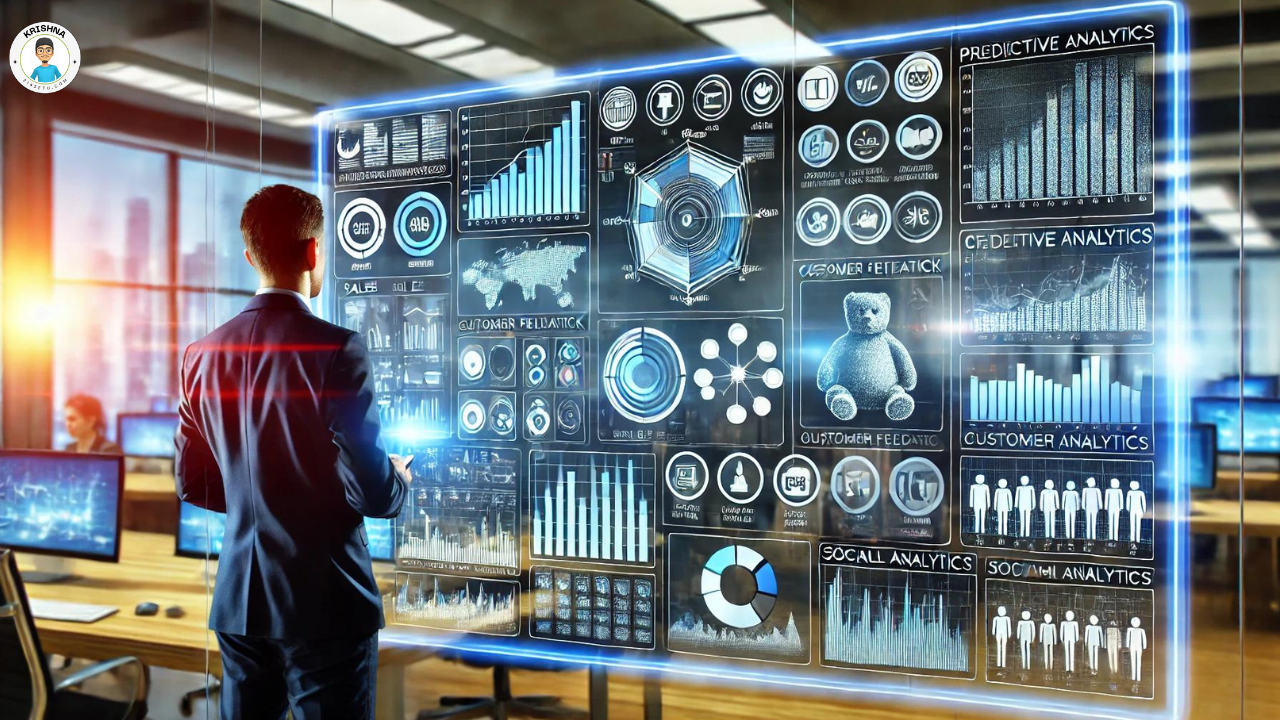
Ever wondered how some businesses seem to know exactly what their customers want? The secret lies in predictive analytics. By analyzing data and making predictions, businesses can understand consumer behavior and tailor their strategies accordingly. In this guide, we’ll explore how predictive analytics can help you understand your customers better and boost your business.
Understanding the Mindset of Your Audience
What is Predictive Analytics?
- Definition:
- Predictive analytics uses historical data, statistical algorithms, and machine learning techniques to identify the likelihood of future outcomes.
- Example: Predicting which products a customer is likely to buy based on their past purchases.
- How It Works:
- Data Collection: Gather data from various sources like sales records, customer feedback, and social media interactions.
- Data Analysis: Use algorithms to find patterns and correlations in the data.
- Prediction: Create models to predict future behavior and trends.
The Importance of Understanding Consumer Behavior
- Personalization:
- Tailor your marketing efforts to individual customers based on their predicted behavior.
- Example: Sending personalized emails with product recommendations.
- Improved Customer Experience:
- Enhance the customer journey by anticipating needs and preferences.
- Example: Offering proactive customer support based on past issues.
- Increased Sales and Loyalty:
- Predictive analytics helps identify opportunities to upsell and cross-sell, increasing sales and customer loyalty.
- Example: Suggesting complementary products during checkout.
Key Techniques in Predictive Analytics
- Regression Analysis:
- Understand the relationship between variables and predict future outcomes.
- Example: Predicting sales based on advertising spend.
- Classification:
- Categorize customers into segments based on their behavior and preferences.
- Example: Identifying high-value customers who are likely to make repeat purchases.
- Clustering:
- Group similar customers together to tailor marketing strategies.
- Example: Segmenting customers based on buying habits.
- Time Series Analysis:
- Analyze data points collected over time to forecast future trends.
- Example: Predicting seasonal sales fluctuations.
- Machine Learning:
- Use advanced algorithms to improve the accuracy of predictions over time.
- Example: Recommending products on an ecommerce site based on user interactions and purchase history.
Tools for Predictive Analytics
- Google Analytics:
- Track and analyze website traffic and user behavior.
- Example: Using predictive metrics to identify potential customer drop-off points.
- IBM Watson:
- Leverage machine learning and AI to gain insights from data.
- Example: Predicting customer churn and suggesting retention strategies.
- Salesforce Einstein:
- Integrate AI-powered analytics with your CRM to predict sales outcomes and customer behavior.
- Example: Forecasting sales pipeline and identifying high-potential leads.
- Microsoft Azure Machine Learning:
- Build and deploy predictive models using cloud-based machine learning tools.
- Example: Analyzing customer sentiment from social media data to predict brand perception.
Implementing Predictive Analytics in Your Business
- Data Collection:
- Gather data from all touchpoints, including sales, customer service, and social media.
- Tip: Ensure data quality by cleaning and validating your data regularly.
- Model Building:
- Develop predictive models tailored to your business goals.
- Tip: Start with simple models and gradually incorporate more complexity as needed.
- Integration and Action:
- Integrate predictive analytics into your existing systems and processes.
- Example: Use predictive insights to inform your marketing campaigns and product development.
- Continuous Improvement:
- Regularly update your models and strategies based on new data and insights.
- Tip: Monitor performance metrics and adjust your approach as needed.
Real-World Examples
- Retail:
- A fashion retailer uses predictive analytics to forecast trends and stock inventory accordingly.
- Result: Reduced stockouts and improved customer satisfaction.
- Finance:
- A bank predicts which customers are likely to default on loans and takes proactive measures.
- Result: Lowered default rates and better risk management.
- Healthcare:
- A hospital uses predictive analytics to anticipate patient admission rates and optimize staffing.
- Result: Improved patient care and operational efficiency.
- Entertainment:
- A streaming service predicts viewer preferences to recommend shows and movies.
- Result: Increased viewer engagement and subscription retention.
Future Trends in Predictive Analytics
- AI Integration:
- Combining AI with predictive analytics for more accurate and actionable insights.
- Example: Using AI to analyze customer sentiment in real-time and adjust marketing strategies instantly.
- Predictive Maintenance:
- Predicting equipment failures and maintenance needs to reduce downtime.
- Example: Manufacturers using predictive analytics to schedule maintenance before a breakdown occurs.
- Behavioral Insights:
- Deepening understanding of consumer behavior through more sophisticated data analysis techniques.
- Example: Retailers predicting not just what customers will buy, but why they make certain purchasing decisions.
Conclusion
Predictive analytics is a powerful tool that can transform how you understand and engage with your customers. By leveraging data to predict future behavior, you can personalize your marketing, enhance customer experiences, and drive business growth. Start exploring predictive analytics today and unlock the full potential of your consumer insights.