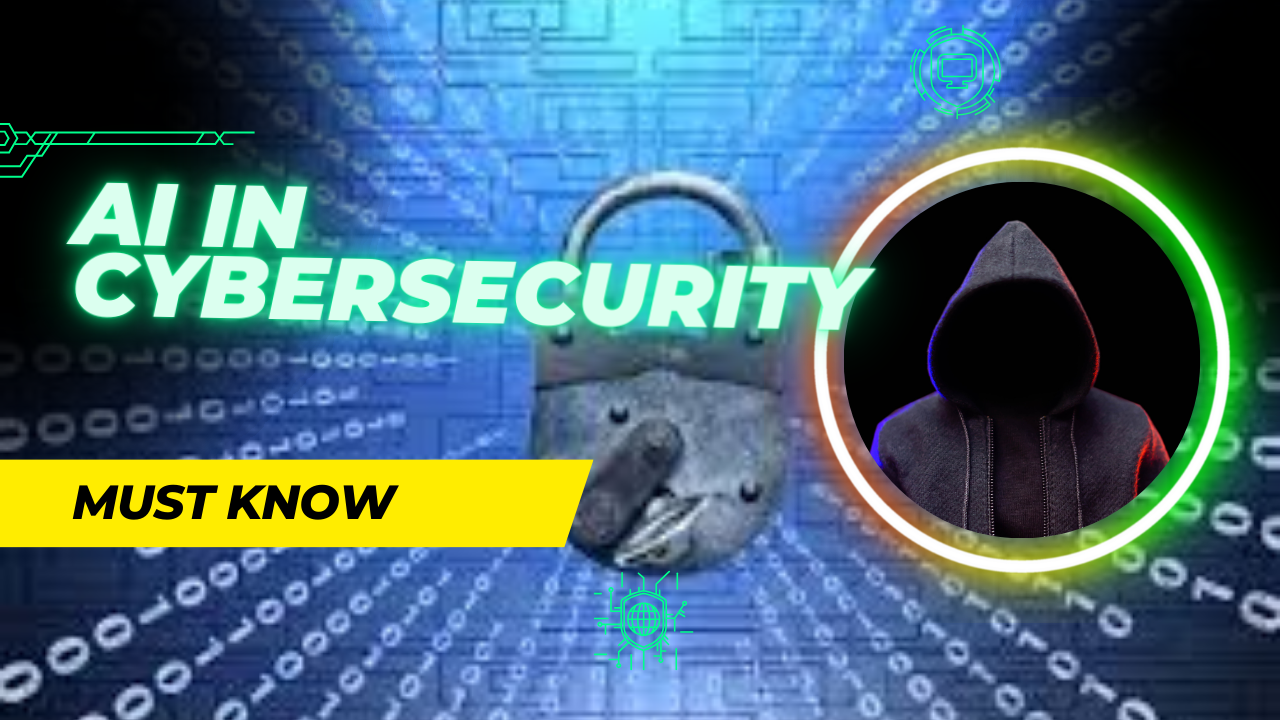
In the rapidly evolving landscape of technology, the fusion of artificial intelligence (AI) with cybersecurity presents both remarkable opportunities and formidable challenges. As AI systems permeate nearly every aspect of modern life—from healthcare and finance to transportation and home automation—the imperative to secure these systems from cyber threats has never been more critical. This blog explores the vulnerabilities inherent in AI, identifies the types of cyber threats these systems face, and offers practical guidance on how to effectively safeguard AI technologies.
The Vulnerability of AI Systems
AI systems are uniquely vulnerable to a range of cyber threats that can undermine their integrity, confidentiality, and availability. One of the primary vulnerabilities is the risk of data poisoning, where malicious actors manipulate the data used for training AI, leading to flawed or biased outcomes. Another significant threat is model stealing, where attackers replicate an AI system’s algorithms by probing it with numerous queries and analyzing the outputs.
Additionally, AI systems can be susceptible to adversarial attacks, where slight, often imperceptible alterations to input data can deceive AI models into making incorrect decisions. This type of vulnerability is particularly concerning in sectors like autonomous driving and facial recognition, where errors can have serious real-world consequences.
Essential Free AI Skill for Developers to know
Types of Cyber Threats to AI
- Data Poisoning: Manipulating the training data to corrupt the AI’s learning process.
- Model Theft: Stealing the AI model’s proprietary algorithms.
- Adversarial Attacks: Inputting deceptive data to fool AI systems into erroneous outputs.
- Evasion Attacks: Techniques designed to avoid detection by AI-driven security systems.
Understanding these threats is the first step in crafting effective defenses against them.
Best Practices for AI Cybersecurity
Securing AI systems involves a layered approach that incorporates robust data handling, continuous monitoring, and the integration of security at the design phase. Below are some key strategies:
- Secure Data Practices: Ensuring that data used for training AI is accurate, clean, and well-guarded against unauthorized access is crucial. This involves implementing strict access controls and regularly auditing data integrity.
- Robust Testing and Validation: AI models should undergo rigorous testing under various scenarios to ensure they are robust against attacks. This includes testing for vulnerabilities to adversarial examples.
- Ethical AI Design: Incorporating ethics and security by design. This means considering the potential misuse of AI technologies and embedding safeguards against such misuse.
- AI-Specific Security Protocols: Developing new security protocols that specifically address the unique challenges posed by AI technologies.
- Collaboration and Regulation: Engaging with the broader cybersecurity and AI community to share knowledge and co-develop standards and regulations that enhance AI security.
Future Outlook and Innovations in AI Security
The future of AI security looks promising with advancements in machine learning models that can detect and counteract adversarial attacks more effectively. Researchers are also exploring the use of blockchain technology to enhance the security and transparency of AI datasets. Additionally, as quantum computing matures, it could offer new ways to protect AI systems from cyber threats or, conversely, pose new challenges.
The Truth About Fusion of Humans and Robots
Conclusion
As AI continues to advance, integrating comprehensive cybersecurity strategies becomes imperative. By understanding the vulnerabilities and implementing best practices, we can protect AI systems from the myriad of cyber threats they face. This not only ensures the safety and reliability of AI technologies but also fosters trust and confidence in their applications across different sectors.